views
Introduction
Artificial intelligence (AI) is transforming healthcare, from diagnostics to personalized treatment plans. But behind every powerful AI lies a crucial but often overlooked process known as healthcare data annotation. This guide explores everything you need to know about healthcare data annotation, from its importance and types to the tools, challenges, and future opportunities. Whether you're a healthcare provider, medical researcher, or data scientist, discover how annotated data can revolutionize medicine and help train smarter AI/ML models.
Why Is Data Annotation Important in Healthcare?
The success of AI in healthcare heavily depends on the quality of the data it processes. Data annotation ensures that raw medical data, such as MRI scans or clinical notes, is accurately labeled, enabling AI models to "understand" and extract meaningful insights.
Benefits of Healthcare Data Annotation
- Improved Diagnostics
AI models trained with annotated data can identify conditions like cancer, heart diseases, or rare genetic disorders with high accuracy.
- Personalized Treatment
Annotation helps AI analyze patient medical histories to create tailored treatment plans, improving healthcare outcomes.
- Enhanced Operational Efficiency
Hospitals and healthcare providers can use annotated data to streamline operations like patient scheduling, inventory management, and emergency response.
- Breakthrough Research
Medical researchers can leverage annotated datasets to uncover new drug discoveries or define cutting-edge treatment approaches.
Macgence specializes in providing data annotation for training such AI/ML models, enabling the advancements listed above and more.
Types of Healthcare Data Annotation
Different types of data annotation cater to the various needs of healthcare applications:
1. Text Annotation
This involves labeling textual data such as electronic health records (EHRs), patient notes, or drug descriptions. It helps AI extract entities like patient names, symptoms, diagnoses, and treatments. For example:
- Named Entity Recognition (NER): Labeling diseases, medications, or procedures.
- Sentiment Analysis: Identifying emotional tones in psychological assessments.
2. Image Annotation
Used for visual data like X-rays, MRIs, and CT scans. Annotating key areas helps AI models detect tumors, fractures, or other abnormalities. Common techniques include:
- Bounding Boxes: Highlighting tumors in radiological images.
- Semantic Segmentation: Differentiating organs, tissues, and anomalies.
3. Video Annotation
Essential for motion-specific healthcare scenarios like behavior monitoring in neurological disorders or surgical training.
4. Audio Annotation
Helps process spoken data, such as doctor-patient conversations, by labeling key details and converting them to text when needed.
Challenges of Healthcare Data Annotation
Despite its importance, healthcare data annotation comes with its own set of challenges:
- Data Privacy and Security
Protecting sensitive patient data is paramount. Regulations like HIPAA must be adhered to, adding complexity to the annotation process.
- Domain Expertise
Not all annotators can robustly label medical data. Adequate training or collaboration with medical professionals is often required.
- Data Complexity
Healthcare data is vast, unstructured, and multifaceted. Handling different formats of data (images, audio, text) can be challenging.
- Cost and Time
High-quality annotation, especially in specialized fields like radiology, is resource-intensive.
Tools and Technologies Used in Healthcare Data Annotation
Numerous cutting-edge tools and technologies are used for healthcare data annotation. Here are some commonly used platforms:
Annotation Tools
- Labelbox
A versatile platform for multiple data types, ideal for medical image and video annotation.
- SuperAnnotate
Tailored for annotating complex datasets, often used in advanced image analysis.
- Lionbridge AI
A scalable solution offering both automated and human-powered annotation services specialized for healthcare.
At Macgence, we combine state-of-the-art tools with domain experts to deliver accurate and scalable healthcare data annotation services.
AI-Powered Techniques
- Natural Language Processing (NLP): Extracts and labels meaningful patterns from textual medical records.
- Machine Learning Automation: Speeds up repetitive annotations, leaving humans to focus on complex cases.
- Cloud Platforms: Scalable data storage and computing, ensuring vast healthcare datasets are seamlessly processed.
Best Practices for Healthcare Data Annotation
To ensure high-quality annotations, consider implementing these best practices:
- Collaborate with Domain Experts
Work with medical professionals to ensure accurate labeling of specialized data like clinical notes or radiology images.
- Automate When Possible
Leverage ML-driven annotation tools for repetitive tasks to save time and resources.
- Prioritize Data Security
Always use secure systems and follow healthcare regulations like GDPR or HIPAA to anonymize sensitive patient data.
- Validation and Quality Checks
Integrate multiple rounds of review to maintain annotation accuracy and consistency.
- Tailored Training
Ensure annotators have deep training in the specific medical domains they work on.
The Future of Data Annotation in Healthcare
The next wave of healthcare innovation will heavily rely on advances in data annotation. Here are some trends to watch:
- AI-Assisted Annotation
Emerging tools will combine human expertise with machine learning to speed up the annotation process.
- Federated Learning
AI models will train on decentralized annotated datasets without exposing sensitive patient information, bolstering data privacy.
- Integration with Wearables
The surge of health data from wearables like smartwatches will necessitate scalable annotation for real-time insights.
- Personalized AI
Annotation will become increasingly patient-specific, enabling ultra-customized AI-driven care.
By collaborating with providers like Macgence, businesses can stay ahead of these trends by training their AI/ML models with top-tier annotated data.
Building a Smarter Future with Annotated Healthcare Data
Healthcare data annotation is the backbone of the AI revolution in medicine. From diagnostics to personalized treatment plans, annotated data empowers AI to make better decisions and drive efficiency. By addressing challenges and adopting best practices, your organization can successfully leverage this technology for meaningful impact.
Partnering with experienced data annotation providers like Macgence ensures your AI/ML models are trained with high-quality data, setting them up for success.
Curious about how we can help? Explore Macgence’s full range of healthcare annotation services today to unlock the power of your medical data.
FAQs
Q1. Why is healthcare data annotation critical for AI development?
Healthcare data annotation ensures that AI models are trained on accurately labeled datasets, enabling them to make accurate predictions and decisions in healthcare applications.
Q2. How does Macgence help with healthcare data annotation?
Macgence specializes in delivering high-quality, secure, and domain-specific annotations across text, image, audio, and video formats to train AI/ML models effectively.
Q3. What are the compliance considerations during healthcare data annotation?
HIPAA and GDPR compliance are essential. Annotators must anonymize any identifiable patient information to maintain privacy and mitigate risks.
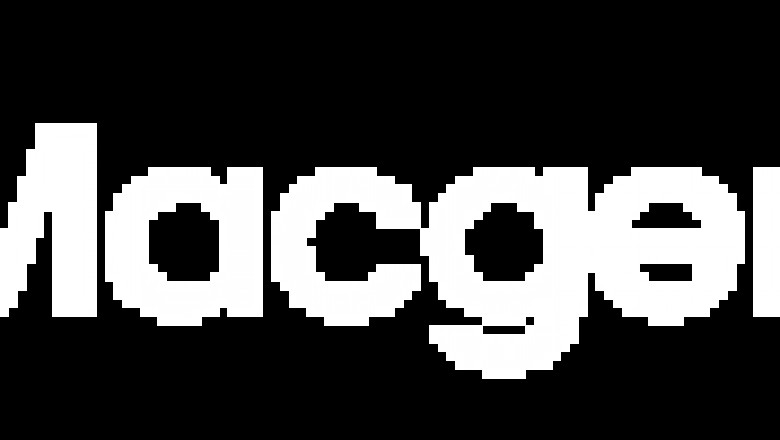

Comments
0 comment